
There was no strong image dependency for univariate scales, but there was for the bivariate schemes. In both the double cone and cylinder schemes, the narrow hue range performed better than the one with wide range. Experiment III showed that the constant hue plane scheme had a better rendering performance than the double cone and cylinder schemes. Experiments I and II demonstrated that the performance of Spectral L* and the three diverging color scales were significantly better than the other six. Experiment II evaluated the scales by having observers judge the values of indicated points in the images. judged the utility of the various renderings. Experiments I and III were paired-comparison experiments in which observers. Experiments I and II tested the ten univariate scales and Experiment III tested the six bivariate schemes. The effectiveness of these color scales was evaluated in three psychophysical experiments. Ten univariate and six bivariate color-encoding schemes were created within the perceptually uniform CIELAB color space. The Color2Gray algorithm is a 3-step process: 1) convert RGB inputs to a perceptually uniform CIE Lab color space, 2) use chrominance and luminance differences to create grayscale target. It shows that dataset A reach 82.55% while dataset B that obtained from KNN-euclidean distance classification on K=5 reach 78.81%. The best accuracy gained from SVM-RBF Kernel on C=40. Here, three classification scenarios are designed: (1) Neural Network-Learning Vector Quantization (LVQ) method, (2) KNN - euclidean distance, and (3) Support Vector Machine (SVM) - RBF Kernel. It identifies the features of PCO and non-PCO follicles based on the feature vectors resulted from feature extraction. It counted by Mean, Entropy, Kurtosis, Skewness, and Variance texture features then obtained 339 follicle images. (2) Dataset B has 40 images consist of 34 normal images and 6 PCOS-indicated images. It counted by Mean texture feature and obtained 275 follicle images.
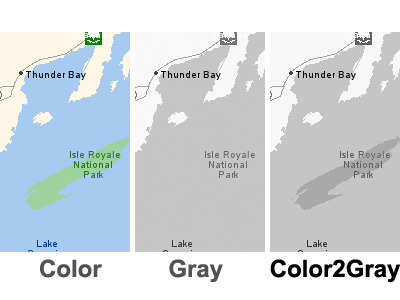
(1) data set A has 40 images that consist of 26 normal images and 14 PCOS-indicated images. This result in 2 datasets prepared for classification process, i.e. The cropped follicle images are categorized into two groups of texture features: (1) Mean, (2) Mean, Entropy, Kurtosis, Skewness, and Variance. The following stage is feature extraction using Gabor wavelet.

The next stage is segmentation with edge detection, labeling, and cropping the follicle images. The first stage of this classification is preprocessing, which employs low pass filter, equalization histogram, binarization, and morphological processes to obtain binary follicle images. This paper designed an application to classify Polycystic Ovary Syndrome based on follicle detection using USG images.
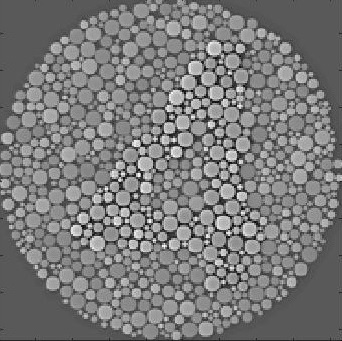
Polycystic Ovary Syndrome (PCOS) is an endocrine abnormality that occurred in female reproductive cycle.
